Logic Model Frameworks for Agricultural Success
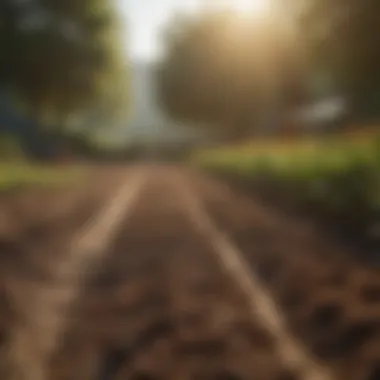
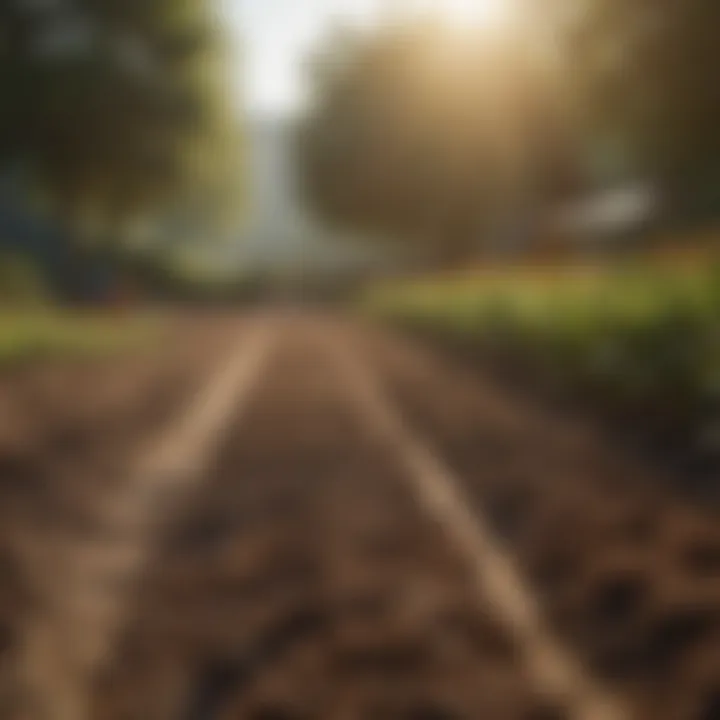
Intro
The agricultural sector faces numerous challenges. These range from climate change to shifts in market demands. In this context, the implementation of logic models can significantly enhance the planning and effectiveness of programs. This exploration aims to provide a structured overview of how logic models function within agriculture, horticulture, and agronomy. By examining real-world examples and theoretical frameworks, one can understand their practical applications in achieving desired outcomes.
Topic Overview
Definition of Key Terms
A logic model is essentially a visual representation. It outlines the relationships between resources, activities, outputs, and expected outcomes of a program. Understanding this concept is fundamental in creating effective agricultural programs. Here are some key terms related to logic models:
- Inputs: The resources required to implement a program. This includes time, money, and personnel.
- Activities: The actions or interventions undertaken to achieve the program goals.
- Outputs: The tangible products or services delivered as a result of the activities.
- Outcomes: The changes or benefits that result from the program, often divided into short, medium, and long-term categories.
Relevance in Agriculture, Horticulture, and Agronomy
The use of logic models in these fields helps stakeholders clarify their goals. For instance, a farmer might aim to increase crop yield through better pest management strategies. A logic model can map out the necessary inputs and outputs to achieve this goal. It also helps identify potential challenges and solutions, allowing for informed decision-making. By applying logic models, farmers and agricultural professionals ensure that their interventions are systematic and data-driven.
Current Trends and Innovations
Recent Advancements in the Field
The agricultural landscape is changing rapidly. New methodologies and technologies are emerging. For example, precision agriculture uses data analytics and technology to optimize field-level management. Logic models can incorporate these new approaches, allowing for more targeted interventions.
Emerging Technologies and Practices
Various technologies are influencing agriculture. These include:
- Drones: Used for monitoring crop health and assessing field conditions.
- Artificial Intelligence: Aiding in decision-making through predictive analytics.
Incorporating these technologies into logic models enables stakeholders to visualize the complex interactions in modern farming.
Practical Applications
Step-by-Step Guides or How-Tos
Implementing a logic model requires a systematic approach:
- Define the Problem: Identify specific agricultural challenges.
- Engage Stakeholders: Collaborate with farmers, researchers, and policymakers.
- Draft the Logic Model: Create a graphical representation of the input-output relationship.
- Evaluate and Adjust: Use feedback to refine the model for continuous improvement.
Tips and Best Practices for Implementation
- Keep it Simple: Avoid overly complex models that confuse stakeholders.
- Regularly Review: Adapt the logic model based on changing conditions or feedback.
- Be Inclusive: Involve various stakeholders to gather diverse insights.
"A well-structured logic model not only clarifies objectives but also enhances accountability between all parties involved."
In summary, logic models serve as a tool to streamline agricultural programs. Their systematic nature encourages thorough planning and evaluation, which is essential in a field confronted by evolving challenges. Through thoughtful application, logic models can drive success in agriculture, horticulture, and agronomy.
Understanding Logic Models
Understanding logic models is essential within the context of agricultural programs. These models serve as a framework that guides the planning, implementation, and evaluation of initiatives aiming to enhance ecological and economic performance in agriculture. By providing a systematic way to conceptualize program activities and their intended outcomes, logic models enable farmers, stakeholders, and policymakers to articulate their visions clearly.
A logic model correlates inputs—resources required for the program—with the activities carried out and the resulting outputs. This structured representation helps identify the causal relationships between actions and results, crucial in agriculture where every decision can impact yield, sustainability, and community well-being.
The benefits of utilizing logic models in agriculture are numerous. First, they facilitate clearer communication among stakeholders. When everyone understands the goals and processes of a program, collaboration becomes more effective. Second, logic models provide a basis for evaluating performance and effectiveness, allowing for data-driven adjustments over time. Furthermore, the use of logic models can help in securing funding by clearly outlining program objectives and projections.
In summary, grasping the concept and construction of logic models is not just advantageous; it is necessary for successful implementation of agricultural initiatives. The clear visualization of how inputs translate into outputs and outcomes fosters accountability and strategic planning, which are both vital in the complex world of agriculture.
Defining a Logic Model
A logic model is essentially a visual representation that outlines the components of a program and how those components interact. It typically includes five main sections: inputs, activities, outputs, outcomes, and impacts. Inputs are the resources, such as funding, personnel, and equipment, needed for the program. Activities detail the actions taken to achieve the program's goals, such as workshops, planting schedules, or pest control measures. Outputs are the direct products of those activities, which could be the number of workshops held or the acreage treated for pests. Outcomes reflect the changes that occur as a result of the program activities, such as improved crop yield or enhanced soil health. Finally, impacts refer to the broader effects of the program on the community, environment, or economy.
This model helps stakeholders understand the logic behind a program's design, providing clarity on how and why specific actions lead to desired results.
Purpose and Importance
The purpose of a logic model in agriculture extends beyond mere planning. It is a strategic tool that emphasizes the importance of aligning resources, activities, and outcomes. By focusing on the flow from inputs to impacts, logic models encourage a holistic perspective on agricultural initiatives. This perspective is particularly critical in an era where sustainable practices must align with economic viability.
Furthermore, logic models are invaluable for ensuring stakeholder engagement. When stakeholders have a clear roadmap of a program’s objectives and processes, they are more likely to support and participate in the initiative.
Components of a Logic Model
Understanding the components of a logic model is essential for anyone interested in agricultural programs. Each of these elements plays a significant role in the overall design, implementation, and assessment of such initiatives. The components typically consist of inputs, activities, outputs, outcomes, and impacts. Examining these components provides insights into how programs are structured to achieve specific goals.
Inputs
Inputs represent the resources necessary to initiate and sustain agricultural programs. This category includes tangible and intangible assets such as funding, personnel, equipment, and materials. Properly identifying and allocating inputs is crucial as they form the foundation upon which all activities are built.
Considering inputs, one must evaluate several factors:
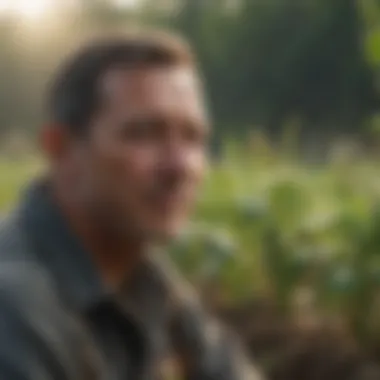
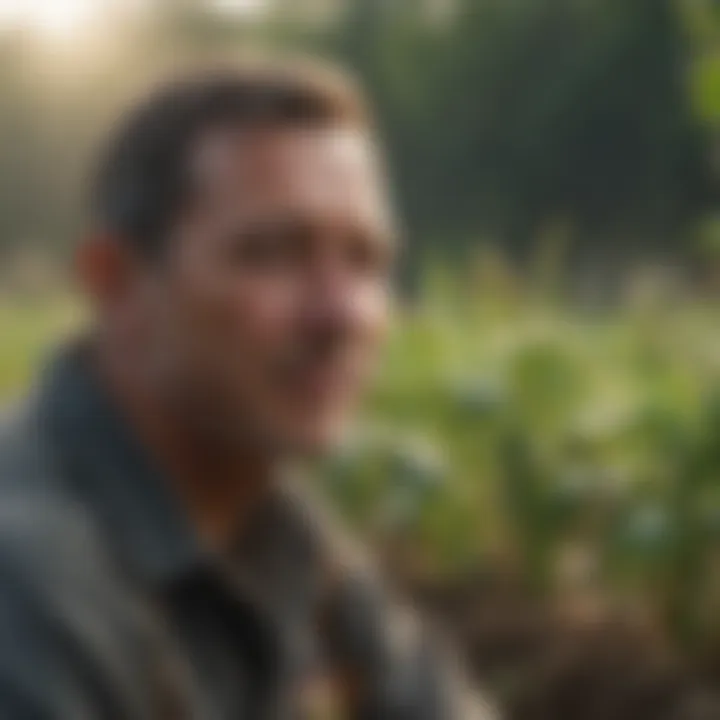
- Funding: Sources can range from government grants to private investments.
- Staff: Expertise and skills of team members can greatly influence success.
- Materials: This covers everything from seeds and pesticides to technology.
By analyzing inputs, stakeholders can assess if they have adequate resources for effective implementation.
Activities
Activities encompass the actions taken to achieve the desired goals of agricultural programs. These are the direct interventions or practices implemented based on the identified inputs. Activities can include training sessions, workshops, field demonstrations, and research initiatives.
Evaluating activities requires focusing on the following aspects:
- Effectiveness: Are the activities designed based on proven best practices?
- Feasibility: Are the activities realistic given the available inputs?
- Innovation: Are new methods considered to improve efficiency?
A thorough examination of activities provides clarity on how well programs are likely to perform.
Outputs
Outputs are the quick results generated from the activities conducted. They are tangible products or services resulting from the implementation efforts. In agriculture, outputs might include completed training sessions, distributed educational materials, or improved farming practices among participants.
It is important to monitor outputs closely because they serve as early indicators of program effectiveness. Key points to consider include:
- Quantity: Number of sessions held, materials produced, etc.
- Quality: Are the outputs meeting the standards set at the beginning?
- Timeliness: Are outputs being delivered on schedule?
Outputs provide crucial data for assessing whether the initial goals of the program are being met.
Outcomes
Outcomes detail the changes or benefits that result from the outputs. They focus on the medium to long-term impacts of the program on the target audience. In agriculture, outcomes might involve increased crop yields, improved soil quality, or enhanced knowledge among farmers.
It is vital to measure outcomes based on:
- Short-term effects: Early behavioral changes resulting from new practices.
- Medium-term effects: Changes in practices and operational processes.
- Long-term effects: Profound impacts on sustainability and economic viability.
Analyzing outcomes allows for a deeper understanding of the program's effectiveness in driving meaningful change.
Impacts
Impacts refer to the broader, long-term effects of a program on the agricultural sector or community as a whole. These may include changes in agricultural policy, environmental benefits, or shifts in local economies.
To fully grasp impacts, consideration must be given to:
- Broad scope: Impacts should transcend the immediate participants and affect wider communities or ecosystems.
- Measurement: Establish clear indicators to track these effects over time.
- Sustainability: Are the positive changes likely to be sustained in the long run?
Types of Logic Models
Understanding the various types of logic models is essential for effectively applying them within agricultural programs. Each type serves a distinct purpose, tailored to different stages of program development and evaluation. Logic models help clarify program intentions, design, and outcomes. They also provide a framework for measuring progress and success. For farmers and agricultural enthusiasts, utilizing the right type of logic model can enhance clarity and improve results, ultimately leading to more effective practices and informed decision-making.
Theoretical Logic Models
Theoretical logic models focus on the abstract concepts behind programs. They serve as a foundation for understanding relationships between components of a program, such as inputs, activities, outputs, and outcomes. These models often draw on existing theories and research in agriculture, allowing for a structured approach to problem-solving.
Key benefits of theoretical logic models include:
- Foundation for practice: They establish a theoretical framework that guides the design of specific programs.
- Guiding principles: They help in understanding the underlying logic that drives program success.
- Knowledge enhancement: These models aid researchers in examining the effectiveness of practices and interventions.
Agricultural practitioners can utilize theoretical models to justify approaches based on robust research, promoting evidence-based decision-making in the field.
Program Logic Models
Program logic models are tangible representations of how agricultural interventions are expected to work. Unlike theoretical models, these focus on concrete actions and results. They describe the pathway from inputs, such as funding or resources, through activities, leading to measurable outputs and desired outcomes.
Benefits of program logic models include:
- Clarity: They present a clear picture of how each component interacts.
- Focus: They keep programs aligned with objectives while offering a basis for accountability.
- Engagement: Stakeholders can better understand their roles and responsibilities within the program.
For farmers, program logic models can help identify the specific actions needed to achieve improvements in productivity and sustainability, ensuring resources are allocated efficiently and effectively.
Evaluation Logic Models
Evaluation logic models are primarily used for assessing the effectiveness of agricultural programs post-implementation. They detail how the evaluation will be carried out, including criteria for measuring success and methods for data collection.
The significance of these models is that they:
- Direct evaluations: They serve as a roadmap for evaluators, outlining what needs to be assessed and why.
- Enhance learning: They facilitate learning by identifying successes and areas for improvement.
- Support accountability: They ensure that stakeholders are held accountable for achieving outcomes.
By employing evaluation logic models, agricultural practitioners can not only measure the impact of their initiatives but also refine their approaches based on evidence.
"Logic models are vital tools in agriculture. They provide clarity and direction, thus driving better outcomes."
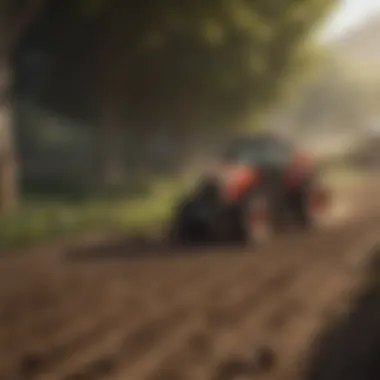
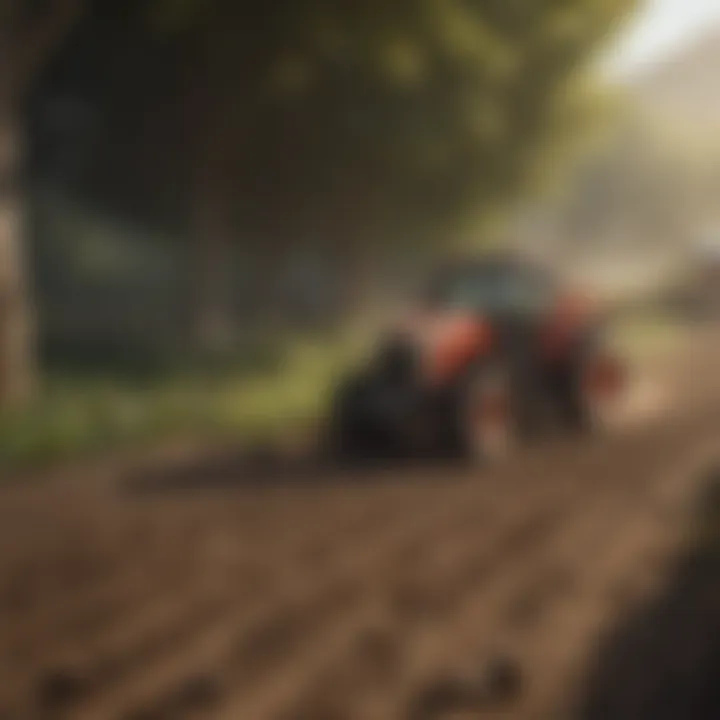
In summary, each type of logic model offers unique insights and implications for agricultural programming. Selecting the appropriate model is crucial for establishing clear objectives and pathways to success.
Logic Model Examples in Agriculture
Understanding logic models specifically within the agricultural sector is vital. These models provide a structured framework, illustrating how elements within agricultural programs connect to their outcomes. By clearly defining inputs, activities, outputs, and outcomes, logic models help stakeholders visualize the pathway to success. They enable program planners and evaluators to assess their initiatives more effectively. The integration of logic models fosters a proactive approach in addressing challenges, ensuring that projects are aligned with broader agricultural goals.
Example One: Sustainable Pest Management Program
Inputs
The inputs of a sustainable pest management program include human resources, funding, and materials like biological pest control agents. These inputs are crucial as they set the foundation for the program's activities. A key characteristic of these inputs is their adaptability; they can be modified to fit diverse agricultural contexts. The unique feature of utilizing biological agents is its eco-friendliness, but it may also present challenges, such as longer timeframes to see results compared to chemical alternatives.
Activities
Activities of this program primarily involve training farmers on pest identification and implementing integrated pest management strategies. The direct involvement of farmers is a beneficial aspect, leading to greater ownership and commitment to outcomes. A unique feature here is the use of community-based workshops, which enables peer learning. However, this method may face limitations in reaching all farmers due to time constraints and varying levels of engagement.
Outputs
The expected outputs from the program include the number of farmers trained and the volume of biological pest control agents distributed. These outputs are measurable, providing tangible evidence of progress. A distinctive feature is the emphasis on collaborative efforts in output generation. Nonetheless, there is a potential disadvantage; not all outputs may indicate positive outcomes if the quality of training varies significantly among participants.
Outcomes
Outcomes reflect the changes in pest populations and crop yields over time. Improved pest management leads to higher quality yields and reduced pesticide use. The measurable nature of these outcomes makes them essential for evaluating program success. The unique aspect of relating outcomes directly to environmental health fosters a stronger connection between agriculture and sustainability. However, long-term outcomes might take time to materialize, creating a delay in perceived success.
Example Two: Soil Health Improvement Initiative
Inputs
Inputs for the soil health improvement initiative include soil testing kits, educational materials, and funding for workshops. These are critical as they equip farmers with the knowledge and tools needed for implementation. A key characteristic is the focus on scientific data, which informs decision-making. One unique feature is the involvement of agronomists in soil testing, ensuring that recommendations are tailored. However, initial costs for inputs can be a barrier for some farmers.
Activities
The activities involve soil testing, workshops, and the implementation of cover cropping and crop rotation practices. These activities are popular since they promote sustainable farming practices. A significant feature is the hands-on nature of workshops, allowing farmers to apply learning immediately. The downside could be the limited reach of these activities, particularly if they are conducted in rural areas with poor transportation.
Outputs
Outputs encompass the number of soil tests conducted and farmers participating in workshops. These outputs provide a clear measure of engagement. The emphasis on tangible outputs is beneficial because it allows continuous monitoring. Nonetheless, focusing solely on quantity may overlook the quality of soil health improvements achieved.
Outcomes
Outcomes include enhanced soil fertility and increased crop yields. These are critical indicators of program success. The unique feature is the potential for outcomes to improve over time, reflecting ongoing commitment to soil health. However, measuring outcomes can be complex, particularly in the face of changing environmental conditions.
Example Three: Crop Yield Enhancement Project
Inputs
In this project, inputs consist of advanced seed varieties, fertilizers, and irrigation systems. These are foundational to achieving the project's goals. A key characteristic of these inputs is their capacity to significantly enhance crop yields. One unique aspect is the incorporation of precision agriculture technologies, which optimize resource use. However, these inputs may demand considerable investment upfront, which can deter smallholder farmers.
Activities
Activities include training sessions on new farming techniques and the implementation of irrigation systems. These are favorable because they improve farmers’ skills and crop management capacity. A distinctive feature is the focus on practical, hands-on learning, which fosters immediate application. However, the challenge remains in maintaining participant motivation throughout the project phase.
Outputs
The outputs of this project should be the number of farmers adopting new practices and the areas planted with advanced seeds. This aspect is advantageous because it allows for easy tracking of progress. That being said, outputs can sometimes misrepresent success if not correctly linked to the achieved outcomes.
Outcomes
Expected outcomes include increased average yields and enhanced farmer income. These outcomes are vital for demonstrating the project's efficacy. A unique feature of these expected outcomes is their potential to create a ripple effect, leading to wider community benefits. However, measuring income increases can be complicated, often dependent on market conditions beyond the farmers' control.
Effective logic models in agriculture are not only frameworks for planning but also tools for evaluation and adjustment in practice.
Using Logic Models for Program Evaluation
Using logic models in program evaluation is crucial in the agricultural sector. They provide a clear framework for understanding how various elements of a program connect and function. Logic models help in articulating goals, strategies, and expected outcomes. They make evaluating effectiveness more systematic.
When stakeholders can visualize the program's flow, they are better positioned to contribute to its success. They understand the pathways to achieving desired impacts. Logic models assist in communicating the rationale behind program decisions and activities. Furthermore, they help identify areas needing adjustments based on feedback and outcomes.
Establishing Evaluation Criteria
Establishing evaluation criteria is an essential first step. Criteria provide benchmarks that inform what success looks like. In agriculture, criteria can include improvement in crop yields, reduction in operational costs, or enhancements in soil health.
When setting criteria, it is vital to:
- Define specific, measurable goals for the program.
- Ensure criteria are realistic given the available resources.
- Engage stakeholders to gain input on what metrics matter.
- Align criteria with broader agricultural objectives to maintain relevance.
By having clear criteria, programs can improve focus and accountability, making it easier to gauge success.
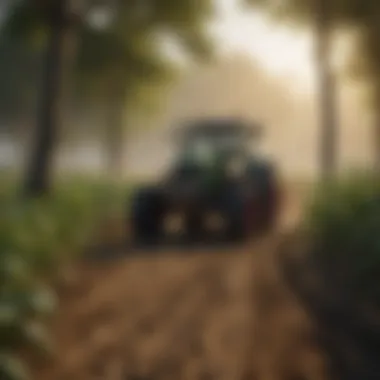
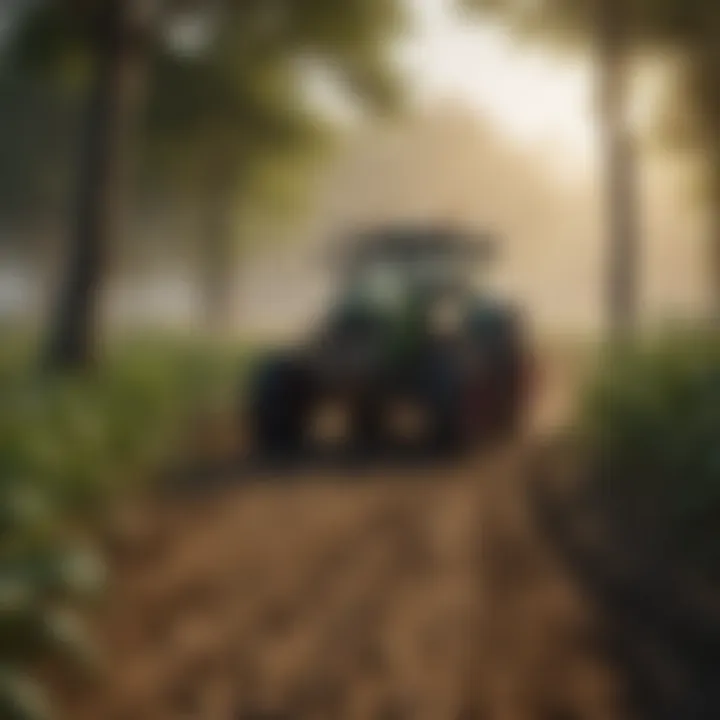
Collecting and Analyzing Data
Collecting and analyzing data is a fundamental component of using logic models effectively. This process involves gathering quantitative and qualitative data that aligns with the established criteria. Sources like surveys, field observations, and production records can be invaluable.
Data analysis allows for:
- A clear picture of both outputs and outcomes of the program.
- Identification of trends and patterns that inform decision-making.
- Understanding what strategies worked and what needs revision.
With rigorous data collection and analysis, stakeholders can adjust practices to enhance program impact.
Making Data-Driven Decisions
Making data-driven decisions is the final piece in the evaluation process. It means using the analyzed data to inform strategies and future actions.
A few key considerations include:
- Regularly revisiting the logic model to incorporate new insights.
- Encouraging a culture of openness where feedback is valued.
- Prioritizing decisions based on evidence rather than assumptions.
"Data-informed decisions lead to more effective agricultural practices."
By applying a logic model in program evaluations, agricultural initiatives can not only track progress but also foster continual improvement and adaptive management.
Common Challenges and Solutions
Addressing common challenges in implementing logic models in agriculture is crucial for achieving desired outcomes. Various projects often run into obstacles that can hinder their success. It is vital to identify these challenges, as understanding their nature allows stakeholders to develop effective solutions. Logic models provide a framework to visualize these obstacles and strategize appropriately. This section aims to outline some specific challenges and suggest viable solutions in enhancing agricultural program success.
Overcoming Stakeholder Resistance
Stakeholder resistance is a prevalent issue across many agricultural initiatives. This resistance can stem from fear of change, lack of understanding, or previous negative experiences with projects. When stakeholders do not support a program, it often leads to poor implementation and eventual failure.
To overcome this, communication is essential. Engaging stakeholders from the initial stages helps build trust. Organizing workshops to educate them on the benefits of the logic model can prove effective. These workshops must not only inform but also empower stakeholders. Their involvement in discussions enables them to share their concerns, which can be valuable for tailoring the logic model to fit their needs.
It is also important to demonstrate quick wins. By showing tangible benefits early in the project, stakeholders may become less resistant and more supportive. For example, if a pest management approach leads to a decrease in crop loss within the first three months, stakeholders are likely to exhibit more trust in the program.
Addressing Resource Limitations
Resource limitations can pose significant hurdles for logic model implementation in agriculture. These limitations may include lack of funding, insufficient manpower, or inadequate technological resources. Without appropriate resources, even the most well-structured logic model cannot achieve its intended impact.
A strategic approach to securing resources is to conduct a needs assessment. Understanding what resources are necessary can guide the allocation process. Additionally, seeking partnerships with local organizations or governmental agencies can provide additional support. Collaboration can foster resource sharing, making it easier to pool available assets and expertise.
Also, embracing innovative solutions can alleviate some resource pressures. Utilizing technology, like mobile applications and data analytics, can enhance efficiency. These tools provide farmers with vital information that can lead to better decision-making without needing large investments in traditional resources.
In summary, addressing these challenges is essential for utilizing logic models effectively in agricultural settings. The right strategies can mitigate resistance from stakeholders and maximize limited resources, setting the stage for successful agricultural initiatives.
Future Trends in Logic Models for Agriculture
The agricultural sector faces evolving challenges and opportunities that necessitate adaptive strategies. Future trends in logic models for agriculture are critical as they can help in the structured formulation of programs that respond effectively to these changes. The integration of innovative ideas and strategies is essential for ensuring that agriculture remains viable and sustainable. As we consider future trends, we will focus on two key elements: the integration of technology and the emphasis on sustainability.
Integration with Technology
Technology is revolutionizing various sectors, and agriculture is no exception. Logic models can significantly benefit from incorporating technology to enhance program implementation and tracking. For instance, mobile applications and data analytics tools can help farmers monitor their practices in real-time, allowing for adjustments based on immediate feedback.
- Digital Tracking: Tools such as precision agriculture can be integrated into logic models for more precise data collection. This ensures that decision-making is based on accurate, up-to-date information.
- Remote Sensing: Technologies like drones enable farmers to gather data over large areas. Incorporating this data into logic models can provide insights into crop health and yield predictions more effectively.
- Collaboration Platforms: Online platforms can facilitate knowledge sharing among farmers, researchers and policymakers, streamlining the communication of strategies and outcomes.
"As technology advances, so must the frameworks we use to evaluate agricultural programs; integrating technological solutions into logic models is a step towards more effective agriculture."
These technological advancements will not only aid in program evaluation but also increase transparency and accountability among stakeholders in agricultural initiatives. Adapting logic models to embrace these innovations is crucial for fostering engaged and informed communities.
Emphasizing Sustainability
Sustainability is an increasingly pressing concern in agriculture. Logic models that prioritize sustainability can help in developing programs that focus on environmentally friendly practices. It is essential to embed sustainable practices in every aspect of agricultural programs.
- Resource Management: Logic models that incorporate sustainable resource management can enhance soil health, protect water supplies, and maintain biodiversity. This leads to more resilient agricultural systems.
- Adaptive Practices: Programs that encourage adaptive agricultural practices, such as crop rotation and organic farming, can be structured using logic models to achieve long-term ecological benefits.
- Community Engagement: Models that promote community involvement in sustainable practices can strengthen local ecosystems and foster a sense of ownership among farmers.
Through the integration of sustainability, logic models can contribute to not just the productivity of agricultural programs, but also to the overall health of the environment. As agriculture progresses, it becomes paramount to cultivate a balance between productivity and the well-being of natural resources.
Epilogue
The conclusion section serves as a pivotal element in this article. It ties together the insights garnered throughout various sections, emphasizing the importance of logic models in agricultural practices. These models offer a structured framework that enhances program planning, execution, and evaluation. The understanding derived from this article illustrates that logic models are not merely academic concepts; they are practical tools that can bridge the gap between agricultural theory and practice.
Reinforcing the Value of Logic Models
Logic models have significant value in the agriculture sector. They provide clarity and direction, which is essential in implementing successful programs. By delineating inputs, activities, outputs, and outcomes, logic models help stakeholders understand the relationship between resources and results. This clarity can enhance collaboration among team members.
Furthermore, logic models aid in identifying key performance indicators. This allows for focused evaluations and objective assessments of program effectiveness. In agriculture, where conditions can be unpredictable, having a clear framework can make a substantial difference in adapting to changes and ensuring sustainability. The use of logic models encourages continuous improvement, fostering resilience and innovation in agricultural initiatives.
Closing Thoughts on Agricultural Practices
As the agricultural landscape continues to evolve, embracing these structured frameworks will only grow in importance. The culmination of efforts highlighted in this article serves as a stepping stone for practitioners, researchers, and policymakers to enhance their programs.
"The application of logic models in agriculture is a step toward fostering transparency, accountability, and effectiveness in every initiative pursued."
By reinforcing the understanding of logic models and their applications, this article aims to guide agricultural stakeholders toward more strategic and impactful practices.